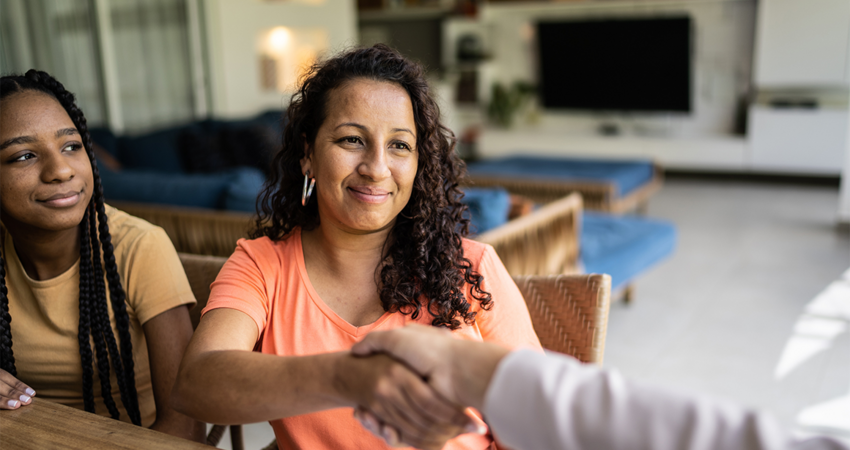
FG Trade, Getty Images
Oversight of Tenant Screening Companies May Change. Here's What Landlords and Renters Should Know to Protect Themselves
Most renters applying for rental housing encounter a tenant screening report. An Urban Institute and Avail survey found that close to 90 percent of landlords are reviewing a combination of a tenant’s income, job history, rental history, evictions, credit history, credit score, and criminal background, increasingly through tenant screening reports.
Landlords seek to minimize their rent and property losses by assessing the reliability of prospective tenants. But the ubiquity of tenant screening reports has increased concerns over errors in the collected tenant data, algorithmically generated risk scores exacerbating racial biases in criminal and eviction records, and faulty name-matching across records. These inaccuracies and errors harm renters by exposing them to the high costs of repeated applications caused by increased denials in the private market and increasing the likelihood of housing instability or homelessness. Data errors also harm landlords by falsely limiting the pool of potential renters and exposing both landlords and tenant screening companies (PDF) to liability for housing discrimination.
Because tenant screening companies don’t share their data management practices or their algorithms, tenants and landlords have very little transparency into the accuracy and quality of the data being used for screening and few avenues to fight inaccuracies.
Agencies such as the Federal Trade Commission, Consumer Financial Protection Bureau, and US Department of Housing and Urban Development worked to protect tenants by filing lawsuits over errors in tenant screening reports and by releasing guidance to hold companies accountable. However, as confusion around staff and funding cuts to these agencies grows, there will be a gap in the oversight and monitoring of these tenant screening practices that affect millions of renters and landlords.
In this new environment, here’s what landlords, renters, and housing advocates need to know about how tenant screening data matching practices may affect them.
How data matching processes and practices may affect tenant screening reports
A recent report from the Urban Institute sheds light into the “black box” of tenant screening by highlighting the challenges and limits of data matching across two components: eviction filings and criminal records.
Using data from Pennsylvania’s unified court system, we worked with the Administrative Office of Pennsylvania Courts to obtain case- and docket-level data on criminal and landlord-tenant case records for 2014 to 2024. To examine how data matching processes affect outcomes, we explored matching data across the criminal and landlord-tenant records along three main dimensions:
- “Unique ID” refers to how different case records within each dataset are attributed to unique individuals. We create three different IDs with varying levels of strictness in what we consider matching records, using name, date of birth, and zip code.
- Match algorithm is how we “score” the similarity of two individuals’ names when combining the criminal and landlord-tenant records using two matching algorithms from the TheFuzz package developed by SeatGeek.
- Match threshold is the numeric cutoff point at which we determine an algorithm’s score to be a match.
Unique Data Scenarios to Compare Unique-Individual Matching Quality Across Datasets
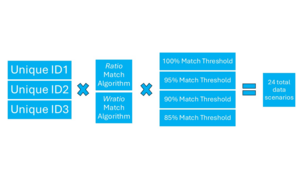
Source: Image created by the authors.
Notes: The image visualizes our methodology exploring different unique identifiers, match algorithms, and thresholds and their impact on who is captured in the harmonized dataset. The unique identifiers range from most conservative to least conservative matching, where “Unique ID1” requires the same name and nonmissing date of birth, “Unique ID2” requires the same name and a missing date of birth that is considered unique only if the zip codes are the same, and “Unique ID3” requires name-only matching. The probabilistic matching algorithms include a ratio scoreand a composite, more lenient wratio score. The various thresholds, ranging from 100 to 85 percent, require different levels of text similarity when identifying a match between the criminal and landlord-tenant datasets.
We use three scenarios to highlight how differences in data practices affect outcomes; ID1 is the strictest approach, and ID3 is the most lenient. Here’s what we found:
The number of people captured varies widely based on data-matching assumptions
We find significant variations in the overall number of individuals captured in the data. The largest variations stem from the “Unique ID,” which changes the total count of people by almost 350,000 between the ID1 and ID3 approaches. This represents a substantial percentage of people within Pennsylvania, suggesting these data practices have wide-ranging impact.
Examining name matches at each threshold level (e.g., 85, 90, and 95) raises important questions about what constitutes a “valid” match (all names in this piece have been changed to preserve anonymity). Setting an appropriate threshold must balance reducing obvious nonmatches between names (e.g., John Doe versus James Doe) without excluding moreambiguous cases that could be argued are valid matches (e.g., David P Smith versus David Paul Smith).
Records associated with people change based on data-matching assumptions
Differences in matching practices also result in large variations in the number of criminal and eviction records attributed to individuals. Under the strictest set of assumptions, a person in our dataset named “Jose Gomez” had a record that included 1 guilty misdemeanor disposition, 1 guilty felony disposition, and 1 eviction filing that was eventually settled. In the most relaxed scenario, the name “Jose Gomez” was associated with 10 misdemeanors, 8 felonies, and 14 evictions, as multiple people’s records are assumed to be linked with one individual.
Similarly, relaxing the match threshold also affects linking tenants across criminal and eviction data. Under the strictest scenario, a person with the name “Cameron Nash” was linked to two guilty misdemeanor dispositions and two eviction filings. When we decreased the name similarity score threshold from 100 to 95, this person was linked to two additional guilty felony dispositions that were associated with the name “Cameron Oliver Nash,” as well as an additional guilty misdemeanor disposition associated with the name “Cameron O Nash,” for a total of three felonies, two misdemeanors, and two evictions.
These variations in middle names, nicknames, and suffixes occur frequently throughout the data, and it’s often ambiguous whether they refer to the same person. Yet, the assumptions made in data matching would have a clear impact on how a landlord viewed the applicant “Jose Gomez” or “Cameron Nash.”
Source-data quality affects outcomes
Our analysis also reveals that data quality—specifically, the presence of key matching variables, such as date of birth—affects matches. The criminal court data have more individually identifying fields with more complete records than the landlord-tenant files. As a result, as the overall population in our dataset declines because of more-stringent consolidation criteria, the estimate of individuals with certain criminal histories stays relatively stable while the estimate of individuals with an eviction filing or eviction disposition drops significantly.
Missing information puts certain people, especially those with common names and those living in large zip codes, at a greater risk of being wrongly tied to records that aren’t theirs in the most-lenient scenarios. Given the known gaps and unreliability in the quality of eviction data nationwide, our results point to the risks of overly relying on landlord-tenant court records for tenant screening without transparent reporting on data quality.
Numbers of Specific Eviction Histories Decrease Significantly as Data Assumptions Are Relaxed
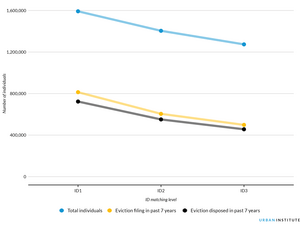
Source: Authors’ analysis of data from the Administrative Office of Pennsylvania Courts.
Notes: ID refers to how records are collapsed within datasets, and each point averages across all data scenarios for that ID. Docket filing date is used to determine the seven-year look-back period for the landlord-tenant data.
What can policymakers, landlords, and renters do to encourage better outcomes for both landlords and renters?
As oversight, regulation, and enforcement may decrease given changes at regulatory and oversight agencies, companies may increasingly use low-quality data practices that harm both landlords and renters. Without federal oversight, landlords, renters, and advocates can still take actions to ensure access to high-quality, reliable reports:
- Increasing transparency in reporting. Landlords and renters can seek to use companies that provide clear information on data sourcing and quality. This includes using companies that are compliant with local and state mandates, such as clean slate laws, and that update their screening criteria to match what’s reliably provided by the screening reports. Additionally, as landlords vet potential tenant-screening vendors, they can ask how prospective tenants’ information is collected and matched across sources to ensure they’re acting on the best information.
- Setting stricter standards for data matches without unique identifiers. Though some data—such as credit score—come with unique identifiers like a Social Security number, most court records, including landlord-tenant and criminal court data, do not. Landlords could consider not using reports that include lower-quality data that lack a unique identifier. Three companies have already stopped using eviction filing data given the data’s problems.
- Assessing data quality and providing guidance on data sources. There is little evidence around which factors in criminal and landlord-tenant histories actually predict lease adherence or high-quality tenancy. As researchers continue to generate evidence on the variables that are most predictive of on-time rent payment and good tenancy, tenant screening companies and landlords can adjust their screening practices and data considered accordingly, especially when many data sources, like eviction filings, have clear limitations.
As questions around the federal government’s role in the enforcement of fair housing laws continue to increase and the role of artificial intelligence and other algorithmic tools in tenant screening grows, greater transparency into this process will be critical for both tenants and landlords.