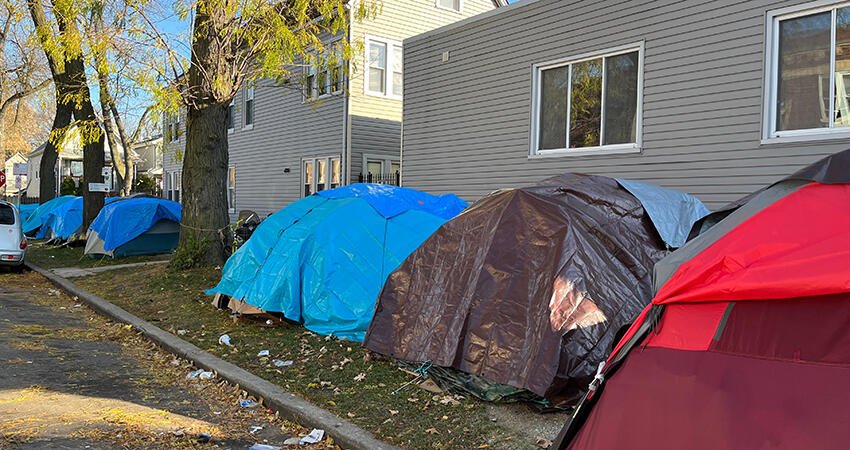
(Pamela Brick/Shutterstock)
Could Machine Learning Help Reduce Inequities in the Homelessness Response System?
This post was originally published on Urban Wire, the blog of the Urban Institute.
As the development of artificial intelligence (AI) and machine learning–driven tools has accelerated, concerns over how tools might drive inequality have also grown. Automated systems can perpetuate biases in data sources and society more broadly, compounding inequities along the lines of race, ethnicity, and gender.
However, machine learning tools also could reduce inequities and improve outcomes for marginalized groups. This could help address homelessness in the US, where systemically racist policies have led Black and Indigenous people to experience disproportionately high rates of homelessness.
In recent years, key data-driven tools in the homelessness field have come under increased scrutiny. The creators of the widely used Vulnerability Index–Service Prioritization Decision Assistance Tool (VI-SPDAT) have recommended that jurisdictions begin phasing out the tool, in part because of equity concerns.
As homelessness researchers and service providers consider how to better target services to those in need, machine learning tools could help advance racial equity in the homelessness response system. Drawing from our analysis of more than a dozen machine learning–driven tools, we highlight two tools driving equitable outcomes in Los Angeles’s homelessness response and offer strategies to reduce bias in tools.
How Los Angeles is using machine learning to reduce bias in homelessness response tools
Few communities have incorporated machine learning tools into their homelessness responses. Among the tools in use, most identify who a community should prioritize for resources. Los Angeles County, California, and the city of Los Angeles, which have some of the largest populations of people experiencing homelessness (PDF) in the country, are at the forefront of developing innovative machine learning tools.
In 2018, the Los Angeles Homeless Services Authority Ad Hoc Committee on Black People Experiencing Homelessness released a report identifying a need to address disparities in how existing triage tools affect marginalized communities. In response, researchers at the University of Southern California and University of California, Los Angeles, (UCLA) led a three-year project to reduce bias in the county’s triage system and increase access to housing resources.
The researchers assessed how well the existing tool, the VI-SPDAT, predicted client vulnerability, and found the VI-SPDAT was more likely to identify white clients as more vulnerable than Black and Latine clients. They then used a machine learning algorithm to improve the tool, training it on Homeless Management Information System (HMIS) and county administrative data. The team also aimed to address bias in data collection, consulting a community advisory board of people with lived experience of homelessness and homelessness service providers. Ultimately, the revised tool reduced bias: the difference in error rate when compared with white clients fell from 5.9 percent to 0.7 percent for Black clients and 3.2 percent to 0.2 percent among Latine clients.
While the triage tool is in the modeling and planning phase, a new homeless prevention program has been launched by LA County in partnership with the California Policy Lab (CPL) at UCLA. Launched in 2021, the Homelessness Prevention Unit reaches out to people identified as being at high risk of homelessness by a predictive model developed by CPL. The model analyzes about 500 factors including emergency room visits, mental health crisis holds, and history of homelessness. The LA County Department of Health Services then contacts those labeled high-risk and provides services that support housing retention. During the program’s first year, a majority of those contacted maintained housing.
Strategies to address racial bias when developing machine learning tools
To avoid perpetuating racial inequities, researchers and practitioners should work to address any potential biases when developing machine learning tools. To do so, they could consider the following strategies:
- Evaluate the validity of machine learning solutions. Researchers and those seeking to implement machine learning tools should first consider common issues that arise when machine learning solutions are used to provide human services. Some research suggests these tools can disproportionately exacerbate racial inequities because the dynamic nature and unobservable elements of homelessness make it difficult to objectively model risk with machine learning systems, even after improving data sources or implementing bias mitigation techniques.
- Use existing data to understand underlying racial disparities in communities. Tools like the US Department of Housing and Urban Development’s Continuum of Care Analysis Tool: Race and Ethnicity, which draws on data from the Point-in-Time Count and American Community Survey, compares the racial distribution of people experiencing homelessness, people experiencing poverty, and the general population. Understanding local disparities can help assess both potential biases in data sources and tool outcomes, such as if they compound existing racial inequities.
- Incorporate debiasing techniques when developing tools. Debiasing techniques often center on equitable data collection, but there’s a growing emphasis on developing methods to address racial disparities in algorithmic tools. IBM’s AI Fairness 360 library provides public source code to help detect biases in datasets and offers resources on these techniques.
Combine different data sources. HMIS data are frequently used for homelessness service provision tools. However, they may have data quality and underreporting issues, especially for some racial or ethnic communities because of stigma and mistrust in the homelessness system and biases in intake questions. Tools could integrate a wider variety of data sources to mitigate potential biases in HMIS data, such as public health data, eviction and land-use data, schools and education systems data, or local public housing agency data.
Additionally, incorporating qualitative data (PDF) through direct feedback is key to developing machine learning tools. Input from people with lived experience, service providers, and caseworkers can provide additional insight and help developers modify their algorithms to drive equitable outcomes.
Research into homelessness response tools that use machine learning is still limited. Those interested in developing these tools should make sure to assemble teams with diverse expertise—including those with lived experience—to identify and address potential biases before machine learning tools are implemented. San Jose’s pilot encampment detection tool has faced backlash from local homelessness outreach workers, who say they weren’t involved in the experiment. As the development of AI tools accelerates, communities should consider how they may reinforce existing inequities.