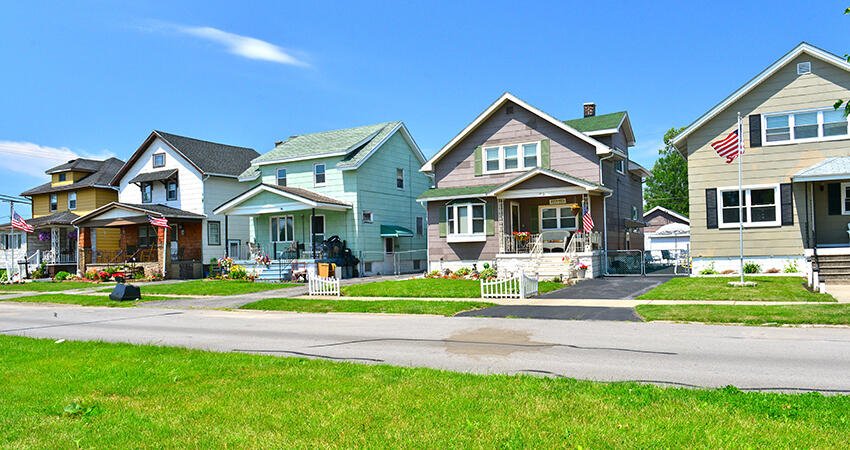
(Richard Cavalleri/Shutterstock)
FinTech Isn’t Enough to End Racism in Mortgage Lending
- Title:
-
New Technology, Old Patterns: Fintech Lending, Metropolitan Segregation, and Subprime Credit
- Author:
-
Tyler Haupert
- Source:
- Publication Date:
-
2022
Financial technology, or “fintech” lending involves a process by which mortgage lenders underwrite loans using all-online applications and proprietary machine learning algorithms on large datasets to assess borrower risk. Research shows that Black and Latinx borrowers at traditional, nonfintech lenders are more likely to be issued subprime mortgages and receive higher-cost loans than white borrowers. Neighborhood-level segregation has also been found to be strongly associated with higher concentrations of subprime lending. By limiting face-to-face interactions between lenders and borrowers, some believe fintech lending could decrease or end racial and ethnic discrimination in the approval rate of mortgage loans and the distribution of high-interest rate subprime loans.
To explore the relationship between subprime lending rates among applicants to traditional and fintech mortgage lenders and metropolitan-level racial and ethnic segregation, the author analyzed Home Mortgage Disclosure Act data from the nation’s 200 largest core-based statistical areas from 2015 to 2017, merged with American Community Survey data on neighborhood racial makeup, homeownership rates, and other neighborhood-level characteristics. They also identified the Community Reinvestment Act eligibility status of each census tract and used two measures of metropolitan segregation: dissimilarity and isolation indices. They calculated these metrics separately for non-Hispanic Black populations and for Hispanic populations (inclusive of any racial group). The dissimilarity index is a measure of racial and ethnic evenness that describes the percentage of a population that would be required to move to achieve a uniform distribution of two racial and ethnic groups across a given area, while an isolation index represents the probability that a person of one racial or ethnic group is exposed to only members of the same group in a given census tract.
The author tested two hypotheses. Hypothesis 1 predicts that, when applying to traditional lenders, applicants from highly segregated metro areas will be more likely to receive subprime loan terms than similar applicants applying from less segregated metro areas. Hypothesis 2 makes the same prediction but for those applying to fintech lenders. From the analyses taken to test these hypotheses, the author also examined the propensity of fintech lenders to offer subprime rates to certain applicants relative to traditional lenders. In this study, a loan was declared as “subprime” if its interest rate at origination was 1.5 points or more above the prime rate reported by the Federal Reserve.
Results show that the probability of an applicant receiving a subprime loan, from both traditional and fintech lenders, was positively associated with metro-area Black and Hispanic segregation. Relative to traditional lending, fintech lending was associated with significantly lower rates of subprime lending in metro areas with high levels of Black segregation.
Key Findings:
- Among traditional lenders, there was a significant, positive relationship between subprime lending and metropolitan segregation across all four measures of segregation (Black and Hispanic dissimilarity indices and Black and Hispanic isolation indices).
- Among fintech lenders, there was a significant and positive association between subprime lending rates and metropolitan segregation, but the relative strength of this relationship compared to traditional lending varied by segregation measure.
- In areas with high levels of white-Black dissimilarity and Black isolation, fintech lenders were associated with lower rates of subprime lending relative to traditional lenders.
- In areas with high levels of Hispanic isolation, fintech lenders were associated with lower rates of subprime lending relative to traditional lenders.
- In areas with high levels of white-Hispanic dissimilarity, fintech lenders were associated with higher rates of subprime lending than their traditional lender counterparts.
- Among all subprime loans originated, relative to traditional lenders, fintech lenders originated subprime loans with larger disparities between Black and white borrowers with similar characteristics, but this borrower-level racial disparity may be explained by neighborhood and metropolitan characteristics.
- Overall, when it comes to subprime lending in segregated metros, fintech mirrors that of traditional lending, if even at somewhat lower rates, depending on the segregation index.
Policy implications
- The author notes that by keeping data and models from regulatory scrutiny, lenders may be violating fair housing legislation. As such, policymakers may want to better regulate fintech lenders through the Community Reinvestment Act or other means.
- As technology, digitalization, and artificial intelligence penetrate consumer finance more broadly, regulators could consider how to identify and minimize potential new biases and ensure consumers remain protected in this new age. This is particularly critical for already marginalized groups, who could face compounding discrimination.
- The transition from face-to-face traditional lending to fintech lending will not end discrimination; regulation and consideration of new manifestations of bias is critical. As the author writes, “Researchers, regulators, and advocates must work to ensure that rapidly evolving technological advances in mortgage lending and other financial processes reduce, rather than perpetuate, racial and ethnic disparities.”